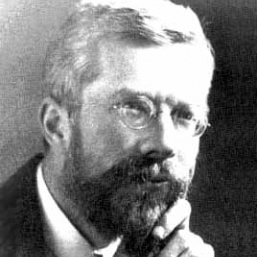
Statistical Methods for Research Workers, 1925
You've probably never heard of Ronald Fisher, one of the founders of modern research methods, but his work and ideas have affected nearly every statistical study -- and the way we understand the world.
Transcript
What does it take for you to be sure? Nobody likes to admit they’re wrong, particularly on any matter of real importance, though no matter how certain you are about something, in the background there’s often that private calculation and the tiniest of doubt.
Eventually, however, there does come a point when it’s just obvious. In lots of cases, our decisions have minor impacts; deciding when you think you’ve made a wrong turn and have to double back, or that maybe that shirt doesn’t go with that jacket after all, are usually forgotten pretty quickly.
For really major stuff — like deciding whether or not to accept the result of a scientific research project — you’d imagine that there was a much more structured and rigorous process to make these decisions. And you’d be right, though the origin of one of the critical components of that process is a bit on the murky side, emerging decades ago in a surprisingly offhand manner. It was likely crystallized, unintentionally, by one of the founders of modern research methods, whose work and ideas you’ve probably never heard of but who has had an effect on nearly every statistical study, and the way we understand the way we understand the world, for nearly a century.
I’m Joe Janes of the University of Washington Information School. Ah yes, statistics, everybody’s favoritest and most fascinating topic. For those of you who haven’t already run screaming, there’s an important story here and I’ll spare you most of the gory numerical details.
Ronald Fisher, by all accounts, was a man of great personal charm and a loyal friend, and also quick to anger especially when he perceived error or misrepresentation in an argument, which led to famous feuds with other early statisticians. He was nearly blind from a young age, married in secret because his 16-year-old wife’s family disapproved, and taught in public schools for a while, though as one source puts it, “neither he nor his pupils enjoyed the experience.” His early work, typical for the emerging world of experimental design, was in agriculture, leading him to interest in genetics and, eventually, eugenics, co-founding the Cambridge University Eugenics Society in 1911.
Here’s the basic idea. Let’s say you wanted to find out whether people have better memory of things they read on paper or on a tablet. You recruit a bunch of people, and have half of them read a chapter of a physical book, and the other half read the same thing on an iPad. You then test their memories for facts in the chapter. Whichever group has the higher score gives you your answer. Simple, yes?
Well, maybe. The higher score might indicate that people in general remembered better in one mode or the other. Or, it might be that the people in one group happened to be better readers or have better memories, or had a natural preference (or hatred) of the format you gave them, or were just having a crummy day. How could you separate any real difference from these other factors? One way would be to randomly assign people to the two groups to mix all those things up. OK, that’s fine, so now you get to this question: how much higher would you want scores for one group to be to be sure that the difference was really because of the format and not just random chance?
That question was very much on the minds of Fisher and his colleagues. A number of ideas were swirling around, as the field was coalescing, using a variety of techniques and guidelines to try to identify what seemed to be the right level of certainty that a result was real. The underlying concepts of statistics are embedded in probability theory, first explored seriously in the 16th and 17th centuries to better understand games of chance, though early concepts date back to ancient dice games using animal bones.
Fisher’s book wasn’t intended to be a textbook, at least not in a classroom setting; it was meant rather to collect, popularize and spread the considerable number of threads of work in statistics which had been developing in earnest for a few decades. It emerged in the same year as The New Yorker, The Great Gatsby, and Mein Kampf, the Scopes Trial, Art Deco, Mount Rushmore, and an August march of 40,000 KKK members down Pennsylvania Avenue in Washington.
Ultimately this question was framed as how much likelihood, what probability of a result being wrong, we should be willing to live with. In the midst of this, along comes Fisher, who seemingly tosses this off on page 79: “in practice we…want to know…whether or not the observed value is open to suspicion…. We shall not often be astray if we draw a conventional line at .05….” A year later, in another paper, he reinforces this: “Personally, the writer prefers to set a low standard of significance at the 5 per cent. point, and ignore entirely all results which fail to reach this level.” Modern researchers would refer to this as a p level of .05, a 5% probability that a research result doesn’t indicate a real effect but rather comes from some random source.
If that sounds a bit casual to be the lodestone of the means by which we decide what studies to believe and which ones we don’t, you’re not alone. There’s no evidence that Fisher intended this dictum to be universal or definitive or exclusive, but there it is. Indeed, if you look a bit more deeply, he does provide a bit more context. The 1926 paper continues: “A scientific fact should be regarded as experimentally established only if a properly designed experiment rarely fails to give this level of significance.” Yet here we are. In the vast majority of settings, if you want a quantitatively based science or social science research study published, and you don’t hit that 5 percent mark, it’s an uphill battle. Moreover, many won’t even submit such results for publication, since who wants to be seen as a failure?
Important consequences emerge from all this. First of all, if you have a hard and fast rule, it’s hard and fast, so a study result that just makes it across the line, winding up with a p value of .0499, gets to be called “statistically significant,” and one that falls just short, with .0501, doesn’t. It’s quite likely that, without Fisher’s writings, some similar setup would have been developed, but it’s safe to say that this standard has impacted profoundly the development of quantitative research, and who can say how many potentially interesting and valuable findings never saw the light of day because they just missed the mark?
Also, consider this. If each statistically significant result has a 5 percent chance of being spurious, then it follows that 5 percent of all the “statistically significant” results that have been published aren’t real, they’re due to random chance. I hasten to point out that studies in the medical and pharmaceutical fields often have considerably more stringent thresholds, 1 percent or even a tenth of a percent, since health and safety are involved. Still, this means that some small fraction of findings…aren’t findings at all.
Think of that 5 percent as the likelihood of a false positive on a test, or as one often hears in beginning stats classes, the likelihood of convicting an innocent person (“beyond a reasonable doubt”). We know these happen, and we want to minimize those chances, and yet you don’t want to require so much certainty that you wind up missing something potentially interesting. This balance between screening false positives and false negatives is very important to researchers, and the focus on p values reveals how they feel about it. There’s a firm line on false positives, and yet in many cases there’s no calculation or even consideration of the probability of a false negative, of failing to see a real effect. The scholarly community wants to be sure that results in the literature are credible, sometimes to the exclusion of results that might otherwise be found.
It could, however, go the other way around. Today, some researchers are increasingly of the opinion that this significance level business is less important than a focus on how big an observed effect is; if people remembered things twice as well when reading from a book than an e-book, that might be worth paying attention to, rather than a smaller effect we’re more sure about. That would give us a different kind of scholarly record, and would require a different way of reading and thinking about and navigating the literature, perhaps with a greater desire and necessity for replication, and the accompanying effort and money and time that would require.
Neither approach is inherently better; they’re just different. You could even imagine combining the two somehow, factoring in the size of effects and certainty in some hybrid way. Is that possible? Could it happen? Getting to agreement on something like that would likely take more than just a line or two in a book, so it would appear that here, as in so many cases, the only thing we can truly be certain of is…maybe.