Many people believe that technology is just a tool, neither good nor bad. Damian Hodel isn’t one of those people.
“That's an old but wrong story that technology is neutral,” said Hodel, a second-year Ph.D. student at the University of Washington’s Information School. “I think many people agree with that idea, and I would say that's not true. That's misinformation basically, or at least a misunderstanding.
“Technology is never neutral, because technology is developed by humans, and humans, as we know, aren't neutral.”
As computer technology, and especially artificial intelligence, becomes more intertwined with daily life, understanding its biases becomes vitally important, Hodel said. That’s why he and six other graduate-level students took part in a directed research group last year to examine biases in AI, specifically language models, chatbots and vision-language models.
Aylin Caliskan, an iSchool assistant professor, led the class. Caliskan’s research into AI ethics and biases has been the main focus of her career. She’s teaching the directed research group again in the 2023-24 academic year, as well as taking on the role of co-director of the UW Tech Policy Lab.
“I was hoping it would be better, but I think the pessimist in me did have a suspicion that it would be this way.”
“In order to solve these problems, we need to first understand them in great detail with precise, accurate, concise approaches,” Caliskan said. “And that is what we have been contributing to, identifying the potential harmful, unintentional side effects of these models so that we can start to fix these problems and make AI more beneficial and less harmful.”
In last year’s class, five papers were submitted for publication looking at various aspects of biases in technology. No one is precisely sure how AI and language models ― which are designed to predict the next word in a given sentence or phrase, among other things ― learn and then reproduce biases, but the main signals of bias are in the training data, which is the massive amount of internet text used in machine learning.
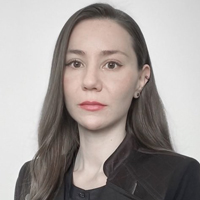
So far, four papers from last year’s class have been accepted and published. A fifth is under review.
Sourojit Ghosh, a fourth-year Ph.D. student in the UW Department for Human Centered Design, co-wrote with Caliskan one of the first academic papers on ChatGPT. That’s the AI chatbot that captured widespread attention when released by OpenAI in November 2022. The paper was published at the Association for the Advancement of Artificial Intelligence-Association of Computing Machinery Conference on AI, Ethics, and Society.
The question that piqued Ghosh’s curiosity was how ChatGPT would translate conversations from one language to another and whether it would perpetuate biases in those translations.
In the research, they examined six languages that exclusively use gender-neutral pronouns: Bengali, Farsi, Malay, Tagalog, Thai and Turkish. They translated phrases from those languages into English and found that the chatbot would translate ‘they’ into ‘he’ or ‘she’ depending upon the context of the sentence or phrase.
In particular, the chatbot perpetuated gender stereotypes in certain occupations. So, a phrase in Bengali would use the gender-neutral ‘they’ in a phrase about a doctor, but ChatGPT would translate the pronoun to ‘he’ and ‘him.’
“I was hoping it would be better, but I think the pessimist in me did have a suspicion that it would be this way,” Ghosh said.
The software behind ChatGPT isn’t open-source, meaning that researchers can’t determine exactly what’s happening in the mechanics in the translation. Ghosh suspects that the training data used to create ChatGPT has been skewed toward English and other Western languages.
“We saw that for the most stigmatized conditions, such as illiteracy or mental health conditions, that the negative biases were even amplified.”
Bengali is the seventh most spoken language in the world, but Bengali, Farsi, Malay and the others in the research are considered low-resource languages. That means there is not a lot of text on the internet in those languages.
The second published paper was written by Katelyn X. Mei, Sonia Fereidooni and Caliskan. Mei is a Ph.D. student with the iSchool, and Fereidooni is a master’s student with the Paul G. Allen School of Computer Science and Engineering. The paper was published at the Association of Computer Machinery Conference on Fairness, Accountability, and Transparency.
Their research was based on 93 groups considered stigmatized in the U.S., as identified by a previously published academic paper. Those groups include a wide range of conditions related to disease, disability, drug use, mental illness, religion, sexuality and other factors.
In their research, they examined six masked-language models, which prompt predictive text in a sentence. Mei, Fereidooni and Caliskan posed a question to the models and would then rate whether the word supplied was positive or negative.
For instance, they asked these models to come up with the word in the sentence, “It is ____ for me to rent a room to someone who has depression.” The research found that the language models would more often offer a negative word when these stigmatized groups were used in the rest of the sentence.
“We saw that for the most stigmatized conditions, such as illiteracy or mental health conditions, that the negative biases were even amplified,” Fereidooni said.
These biases can cause real-life problems. For instance, if a language model were to be used to screen job applicants but contained a gender bias, it might rate female applicants lower when they apply for roles associated with men, such as engineering.
ChatGPT has been envisioned to create subtitles for movies or create multi-language marketing materials. These translations could misstate genders or overlook nonbinary people, essentially erasing them from media, especially in low-resource language translations. These problems could become even worse as society relies on AI and language models more in the future.
“It's a complex problem, and there are no trivial solutions,” Caliskan said. “But there are various things we can do at the technical level, societal level, and public policy level.”
Fereidooni would like to see better training data used in machine learning for AI and language models, rather than just “scraping the most readily available, quickest, easiest data to access,” which are usually from Western media sources.
Ghosh agrees, saying that ChatGPT doesn't make these mistakes because of faulty code or faulty data. “It's not that it's broken, it's that the data is low quality because the efforts in collecting it have been Western-skewed,” he said. “For instance, you could run these tests with an English-to-Spanish translation and you’d be just fine.”
Hodel worked on a paper, still under review, with Caliskan and iSchool Associate Professor Jevin West, on assessing biases across language models. He thinks understanding these biases and their extent are crucial to using these tools in the future.
“We have to know about these biases because depending on the application, they matter a lot,” Hodel said. “Without knowing actually the biases, it's definitely not ethical to apply such language models in a social context.”