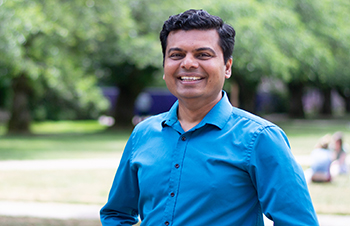
Can our search results be fairer? Can the systems that generate them be smarter? Are the recommendations we get from Google, Amazon or Netflix really the best they can be?
Those are some of the topics researchers investigate in the Information School’s InfoSeeking Lab, which works to make search and recommendation systems fairer, more diverse and more transparent.
The lab migrated from Rutgers University to the University of Washington with its director, Associate Professor Chirag Shah, when he joined the iSchool in 2019. It recently celebrated its 10-year anniversary — a decade in which it has generated highly cited research, produced 14 Ph.D. graduates and garnered more than $4 million in funding.
When users search online, they don’t just deal with a neutral machine; they encounter recommendations that are produced by algorithms and personalized to appeal to them. Those systems contain baked-in biases, Shah said, such as promoting sensationalized stories because they generate more clicks than reliable sources of information. In one of the lab’s Responsible AI initiatives, its FATE (Fairness, Accountability, Transparency, Ethics) Group is studying ways to address these biases and increase fairness and diversity among search results.
In a recent experiment, members of Shah’s research team tested whether they could improve the diversity of Google search results without harming user satisfaction. They replaced a few of the first-page results with items that would normally appear farther down the list, offering different perspectives. They found that users were just as satisfied with the altered results.
“Diversity of information is easy; doing it the right way is hard.”
“If we can sneak in things with different views or information and people don’t notice, then we have improved the diversity,” Shah said. “We have given them more perspectives without them feeling like we have toyed with their satisfaction.”
But Shah wondered if there might be a dark side. If users couldn’t distinguish between two sets of search results, would the same principle apply to misinformation? To find out, researchers in the lab ran a similar experiment where they sprinkled debunked COVID-19 related misinformation into search results. They again found that people couldn’t tell the difference.
The two experiments illustrated the challenge of diversifying search results. A well-intentioned effort to offer users a broader range of sources could inadvertently bring misinformation to the fore, and if that misinformation drew a lot of clicks, it would teach the algorithm to favor it on subsequent searches. Meanwhile, suppressing less-trusted information can open up search engines to charges of bias.
“Diversity of information is easy; doing it the right way is hard,” Shah said. “There’s a whole community working on bias in search and a whole community working on misinformation. This is the first time we’ve shown how one affects the other.”
Outside the ‘black box’
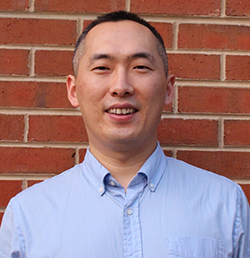
Another InfoSeeking Lab initiative aims to increase the transparency of search results and give users more information about why they’re seeing what’s being recommended to them. Most users know that when they perform a keyword search in Google, a hidden algorithm spits out customized results. But Google doesn’t disclose the data it uses to tailor those recommendations or tell users anything about what produced that set of links.
“The idea is to create explanations because a lot of recommender systems are just a black box,” Shah said. “If you want to earn users’ trust, if you want to create a fair system, you need to be able to explain your decision process.”
As technology makes systems smarter, it can become more complex at the expense of transparency and fairness. Shah hopes to show that greater transparency can be good for business, and he wants to educate users so they’ll demand to know more about the reasons behind the recommendations.
“When you’re trying to make systems smart, it often means making them more opaque and less fair,” Shah said. “My hope is we get to a place where you’re building AI systems that you want to make smart and you also want to make them fair, and these are not competing goals.”
Postdoctoral scholar Yunhe Feng is applying that lens of fairness to research on Google Scholar, a widely used search engine for scholarly literature that relies on keyword searches.
“Google Scholar just picks up the most relevant documents and returns them to users, but you can’t say they’re the fairest,” said Feng, who joined the iSchool this summer after completing his Ph.D. at the University of Tennessee.
Feng is developing a technique to rerank the results after accounting for more information about the research, such as its author’s gender or national origin. By changing the results to distribute them evenly by these factors, his algorithm raises the profile of research that would otherwise be buried deeper in search results.
The concept of fairness is at the heart of the InfoSeeking Lab’s work, but as Shah noted, fairness is a social concept and a moving target. In the 19th century, for example, it was generally considered fair that American women didn’t have the right to vote; and our system of taxation is constantly changing based on what elected leaders consider to be fair at the time. As a result, the lab works to create tools that will enable fairness and let users define it.
“We don’t want to decide what’s fair, but we want to give you the ingredients to say, ‘In this time and context, fairness means this,’” Shah said.
Smarter searching
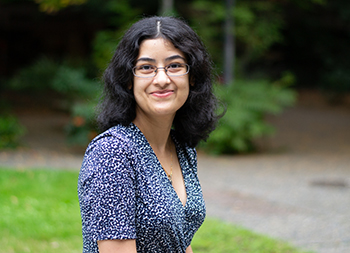
Along with making search and recommendation systems fairer, researchers in the lab also want to make them smarter. Ph.D. candidate Shawon Sarkar said that as sophisticated as search engines have become, they often don’t understand the underlying task when people search. If you type the keyword “Rome,” for example, Google doesn’t know if you want a history lesson or a vacation plan.
“From people’s interactions with the systems and search behaviors, I try to identify the actual task that people are working on,” Sarkar said. “If I can identify that, then I will provide better solutions for them.”
Sarkar became involved in the lab as a master’s student at Rutgers and followed Shah to the UW iSchool to complete her Ph.D. She praised Shah’s mentorship and said she finds encouragement from the lab’s collaborative atmosphere.
“We’re like a family in the lab — both the existing students and alumni. We still keep in touch with all the alumni. They’re very good connections in academia and industry, but also in our personal lives.”
And like others in the lab, she has her eye on the greater good.
“I think my work will help people find accurate information more easily and then make more informed decisions in life,” she said. “That’s the main goal. It’s a pretty big goal!”
Learn more about the InfoSeeking Lab at infoseeking.org.