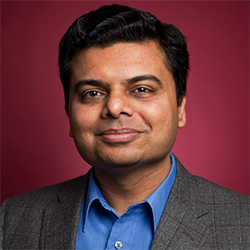
It’s uncanny, and sometimes maybe a little creepy. When you search for something on Amazon or Netflix, the search engine seems to know you — or at least know enough about you to recommend the right brand of tube socks or the right movie.
Something is going on behind the scenes to make that happen, but how much do you know about how those results are generated? And how much do you want to know?
Chirag Shah, an associate professor at the University of Washington Information School, will explore such questions in a three-year research project on explainable recommendation systems. Shah and colleague Yongfeng Zhang of Rutgers University recently received a $500,000 National Science Foundation grant to develop useful ways to improve the transparency of search recommendations.
“There are so many ways to provide transparency to the end user, but many of them are useless,” said Shah, who joined the iSchool this past fall. “It doesn’t help to show the code or the algorithm to a user. Technically you’re being transparent, but most people are not going to understand or care.”
On the other end of the spectrum are systems that make things look overly simple. “When you tell the user, we’re recommending this because people like you also like this, it kind of makes sense, but it’s a half-truth because there’s a lot more going on,” Shah said. “How can we be transparent, yet useful for an average user?”
To find out, Shah will use publicly accessible data from search systems run by companies such as Amazon, Netflix, Spotify and Microsoft. He and fellow researchers will investigate how to layer effective explanations on top of the existing search systems. Their research will show the effectiveness of different explanations based on the task a user is performing. For example, the Amazon user looking for tube socks might want a different level of transparency than someone surfing through movie recommendations or seeking medical advice.
Shah hopes the research will improve the fairness of search recommendation systems by exposing them to more critical thinking from users. When searchers only see what the system recommends, they only see a fraction of the possible choices available to them. This reinforces biases that are often unintentional, but are the result of having a lack of transparency and lack of awareness, Shah said.
“They’re only seeing what’s being recommended. They’re not seeing what’s not being recommended,” Shah said. “People don’t know what they don’t know.”
This project falls under the larger umbrella of research agenda that Shah refers to as FATE (Fairness, Accountability, Transparency, Ethics). The project is scheduled to run through 2022.
Learn more about Shah's FATE research group: