Catch a Cry
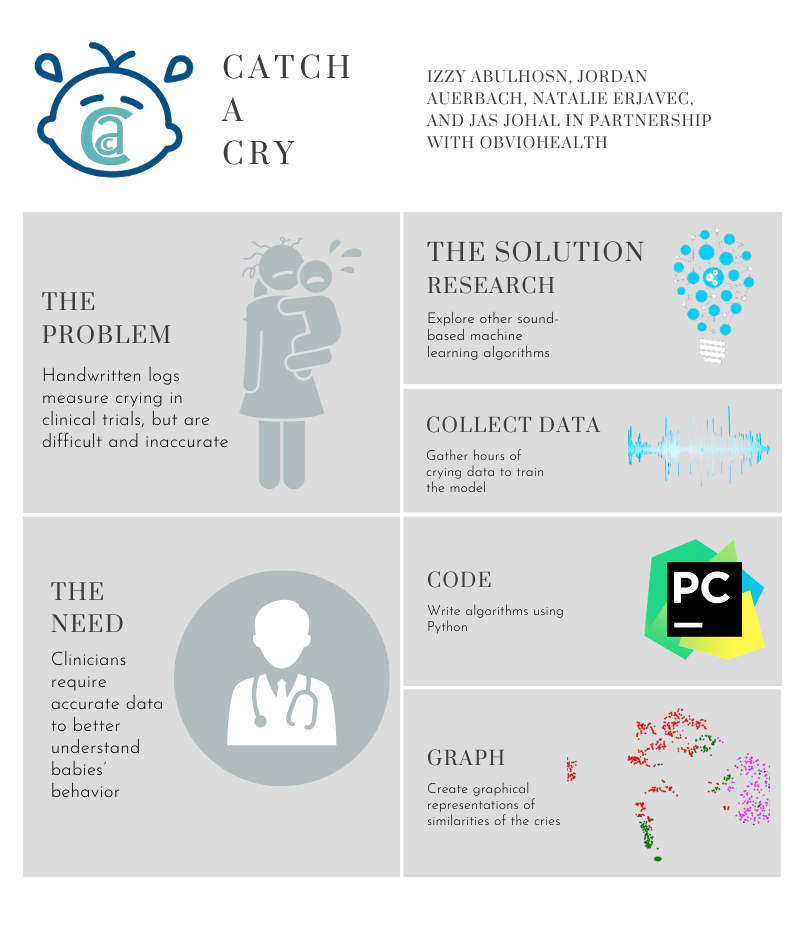
Doctors and parents in clinical trials face significant difficulties in tracking babies’ cries. The current method, handwritten crying logs, is inaccurate and offers little insight for clinical personnel. This project aims to use machine learning algorithms to more accurately identify and track cries. Hours of crying data were gathered and transformed into t-Distributed Stochastic Neighbor Embedding, Support Vector Machine, and k-Nearest Neighbor algorithms, visually grouping audio clips by sound similarity. An audio debugger determines the accuracy of the groupings. With continued training and testing, the algorithms will be able to engage binary classification of cries.
Project sponsored by: ObvioHealth
Project participants:
Natalie Erjavec
Informatics
Izzy Abulhosn
Informatics
Jordan Auerbach
Informatics
Jas Johal
Informatics