Making Sense of Misinformation At Scale
data science & visualization
information policy & ethics
software development
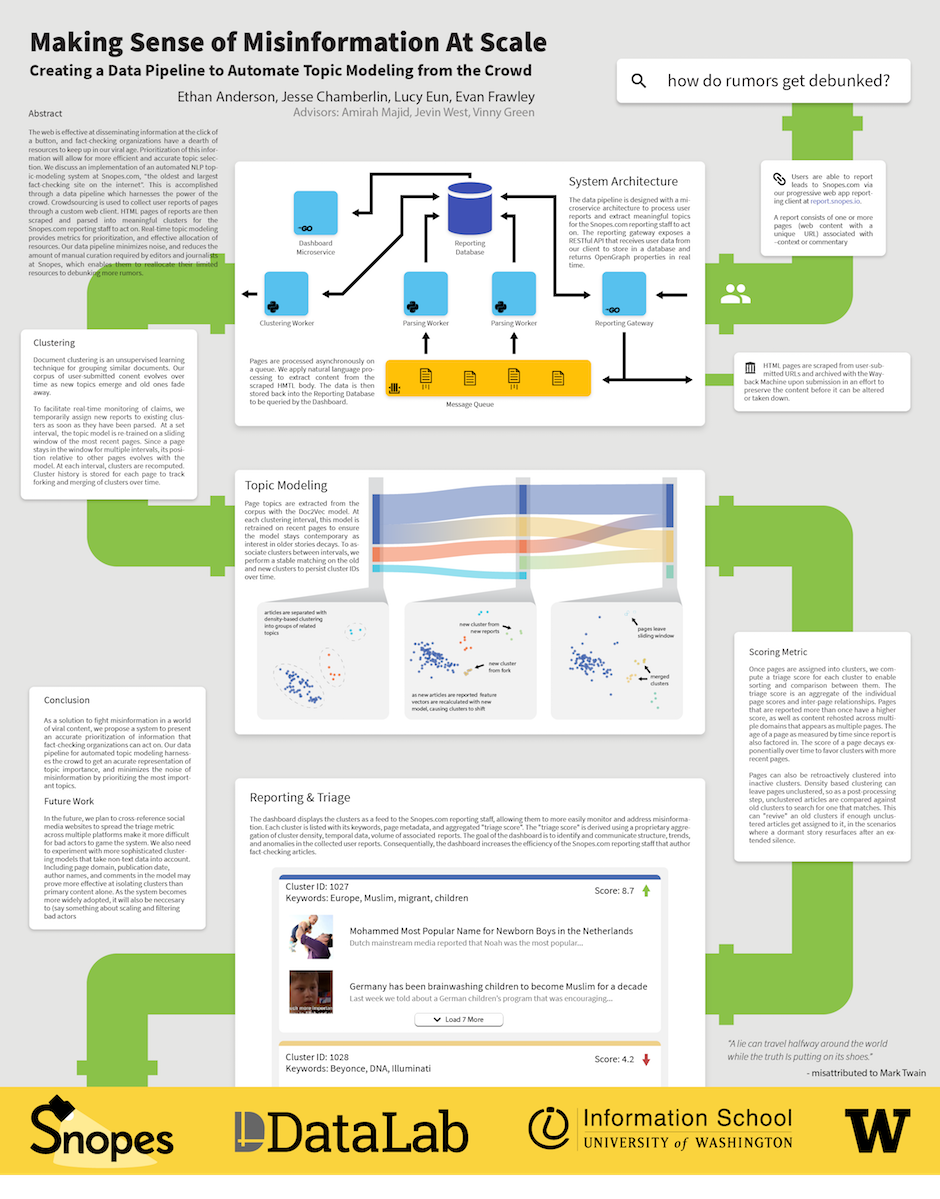
Fact-checking organizations have limited resources to keep up with misinformation in this viral age. Prioritization of information allows for more efficient and accurate topic selection. We discuss an implementation of an automated NLP topic-modeling system at Snopes.com, a data pipeline to crowdsource misinformation. Users submit reports through a website and HTML is scraped and parsed into clusters for the Snopes.com reporting staff to act on. Topic modeling provides metrics for prioritization and effective allocation of resources. Our data pipeline reduces the amount of manual curation required by editors at Snopes, which enables them to reallocate their resources to debunking rumors.
Lucy Eun
Informatics
Ethan Anderson
Informatics
Jesse Chamberlin
Informatics
Evan Frawley
Informatics