Predicting Student Churn
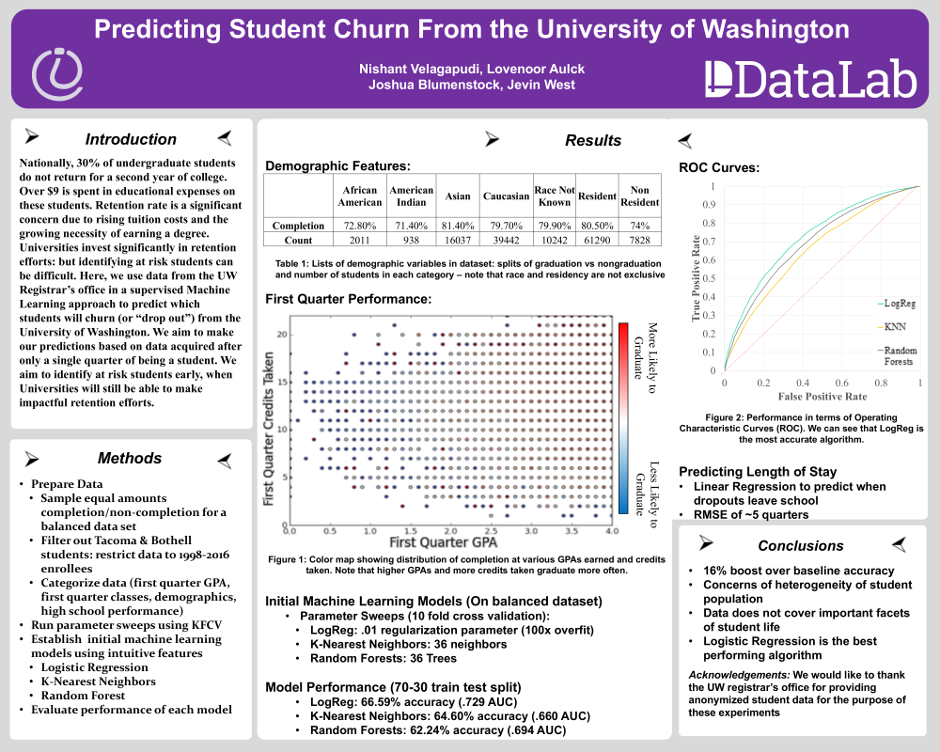
Each year, roughly 30% of first-year students at baccalaureate institutions do not return for their second year and over $9 billion is spent educating these students. Yet, little quantitative research has analyzed the causes and possible remedies for student attrition. Here, we describe initial efforts to model student dropout using the largest known dataset on higher education attrition, which tracks over 32,500 students' demographics and transcript records at one of the nation's largest public universities. Using a balanced dataset, an accuracy of 16% over baseline (66%) can be achieved. Logistic regression, random forest, and k-nearest neighbors models were used. Accuracy was boosted through a feature engineering approach. This project will inspire universities to use machine learning to identify at risk students. They can then target retention efforts towards these students; hopefully putting loan money to better use and ensuring that the most students possible earn degrees.
Nishant Velagapudi
Informatics